GNIFdb Utility
The intrinsic feature of neoantigens include a variety of different amino acid descriptors as well as the physicochemical properties of neoantigens that can reflect their unique role in the immune response. GNIFdb integrates and fully visualizes neoantigen intrinsic feature and other relevant data, allowing users to identify immunogenic neoantigens in different glioma subtypes. therapies. GNIFdb provides an important resource for the development of personalized neoantigen-based therapies. Below, we provide two examples of GNIFdb's use in bioinformatics analysis.
Case study 1: Good prognostic GBMs having neoantigens with protective intrinsic features
Generally, the tumor mutational load of the cold tumor GBM is low. The number of neoantigens and the DAI model (difference in binding affinity between wild-type and mutant peptides) which have valided in other cancer species are not fully applicable for GBM and can predict survival of only a few glioma subtypes. Of the 2928 features provided by GNIFdb, 22 features were significantly associated with survival in IDH WT GBM patients in both TCGA and Asian population data. Of particular note were 12 features that showed strong correlations and all showed protective effects, mainly with the molecular weight and size or volume of the dipeptides comprising neoantigens position 3 and 4, and neoantigens position 2-4.In addition, for patients with IDH WT GBM, the long survival profile is mainly associated with developmental and cell cycle-related GO terms, such as neurodevelopment, renal muscle, and cell cycle. These prognostic intrinsic features of nascent antigens can be manipulated to identify nascent antigens with high potential immunogenicity.
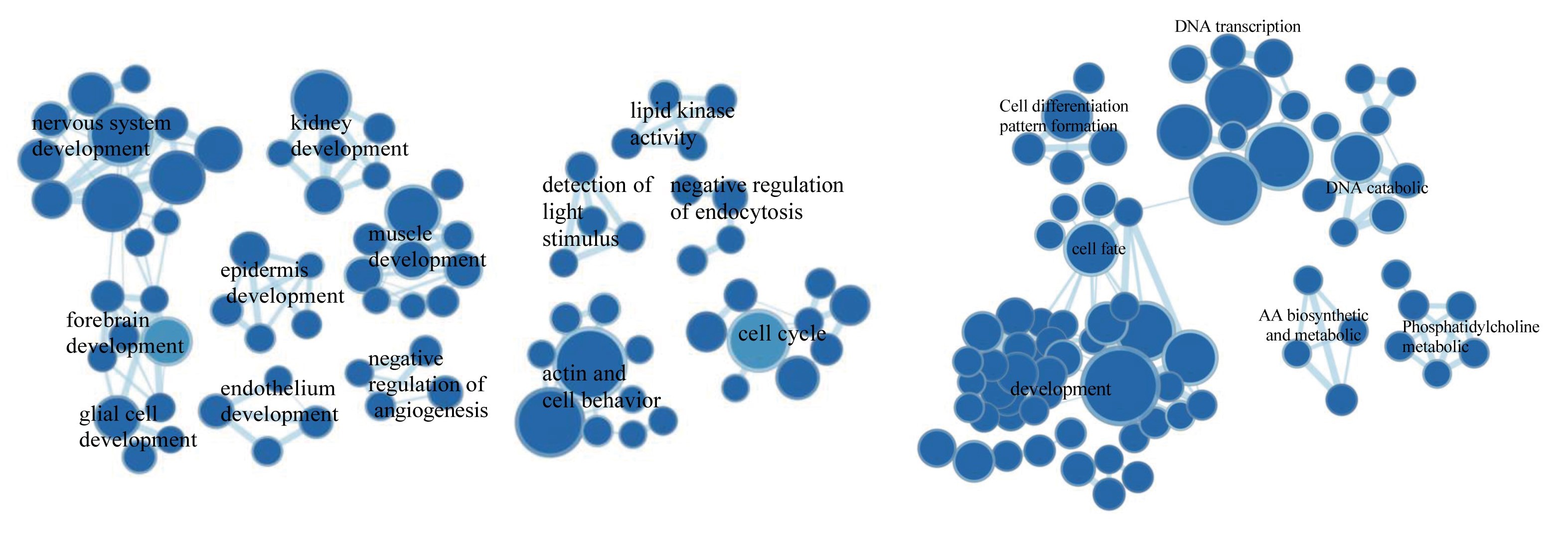
Enriched gene network in GBM
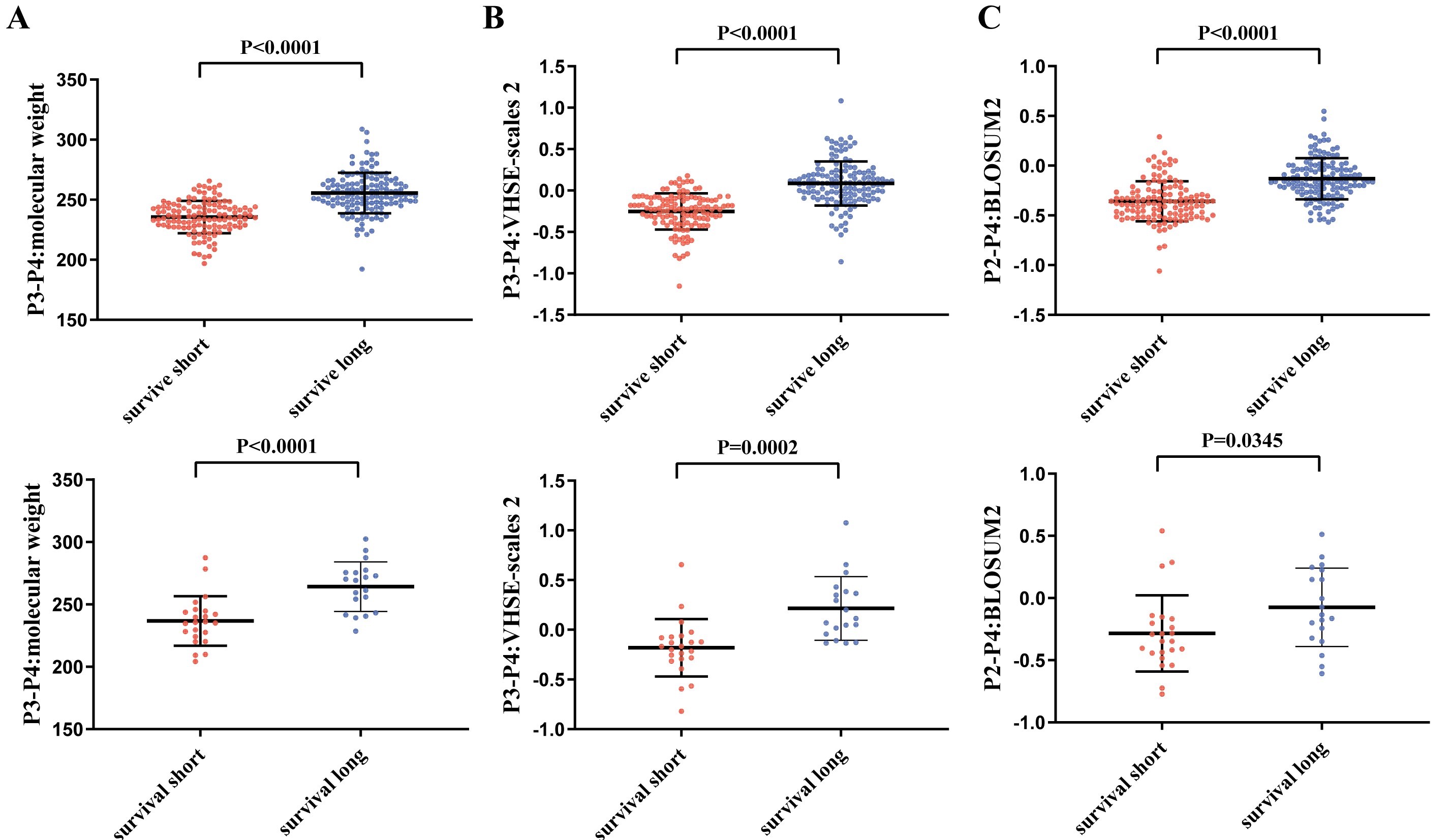
Comparison of feature values between long- and short-term survival IDH wild-type GBMs. A, molecular weight of dipeptide composed with sites 3 and 4. B, VHSE-scales2 of dipeptide composed with sites 3 and 4. C, BLOSUM2 of tripeptide composed with sites 2 and 4.
Case study 2: Neoantigen intrinsic features based deep learning model predicting good prognostic GBMs
Although there have been several studies using deep learning models for survival prediction in cancer patients, fewer studies have focused on survival stratified prediction of patients with GBM. Moreover, the vast majority of glioma prognosis-related deep learning models use gene expression, clinical information and medical imaging data as input features, and no studies have used neoantigenic features to predict patient prognosis for the time being. To identify GBMs with good prognosis and rich in protective intrinsic features, we constructed a deep learning model based on intrinsic glioma features, neoDL. The model was able to successfully stratify IDH wild-type GBMs into two subgroups with significantly different survival rates in both the TCGA cohort and an Asian population cohort, even in some other high-grade glioma subtypes. We compared deep learning models with other data as input to neoDL and found that neoDL classification with intrinsic features as input significantly outperformed DeepLearningModel [1] and PASNet [2] with gene and related pathways as input. This may be due to the fact that neoantigens with immunogenic properties can effectively promote tumor-specific T cell responses and influence antitumor immune responses, thus affecting patient prognosis as well as survival. This suggests that the good results of neoDL in survival prediction are determined by the combination of effective neoantigen features and deep learning networks, which fully demonstrates the great potential of neoantigens in immune prediction and treatment.
[1] Wong KK, Rostomily R, Wong STC. Prognostic Gene Discovery in Glioblastoma Patients using Deep Learning. Cancers (Basel). 2019; 11.
[2] Hao J, Kim Y, Kim TK, Kang M. PASNet: pathway-associated sparse deep neural network for prognosis prediction from high-throughput data. BMC Bioinformatics. 2018; 19:510.
Sun, T., He, Y., Li, W., et al. neoDL: a novel neoantigen intrinsic feature-based deep learning model identifies IDH wild-type glioblastomas with the longest survival. BMC Bioinformatics, 2021.22(1): 382. doi:10.1186/s12859-021-04301-6